#Life2Vec #AI #Machine Learning # Death Calculator #Life Calculator
🤓 관련글 : 위고비와 오젬픽 : 부작용 없이 쉬운 다이어트 ?
😎 이전글 : 2024 미국 대선과 한국 경제의 미래 (feat. 테일러 스위프트)
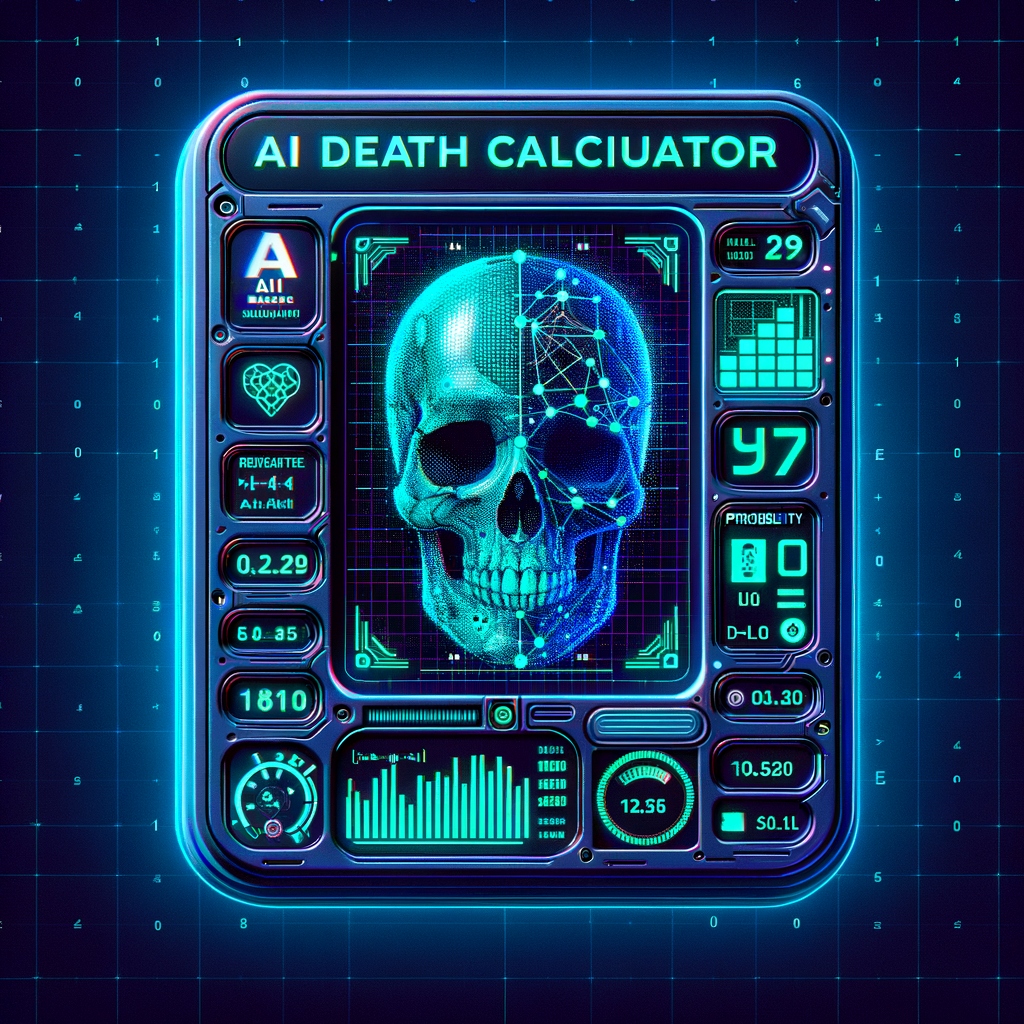
죽음과 삶을 예측하는 AI,
무당도 빅테이터라더니…
인간의 삶을 수치로 나타내고, 그것을 바탕으로 미래를 예측한다면 어떨까요? 철학적이거나 비과학적인 이야기가 아닌, 실질적인 현실로 다가온 것이 바로 머신러닝과 데이터 분석의 발전입니다.
오늘날, 디지털 시대에 우리의 삶은 점점 더 문서화되고 기록되고 있습니다. 온라인 활동부터 금융 거래까지 매일 방대한 양의 데이터가 생성됩니다. 공공 부문에서도 교육, 건강, 고용 등에 관한 정보를 수집하고 저장하는 등의 노력이 이뤄지고 있습니다. 건강 데이터를 활용한 예측 모델에 많은 관심이 기울여져 왔고, 미래 결과를 예측하기 위해 더 넓은 삶의 이벤트를 활용하는 노력이 커지고 있습니다.
머신러닝을 통한 삶의 이벤트 예측
최근 연구에 따르면, 최근 연구는 수많은 다양한 데이터셋을 사용하여 훈련된 AI 변환 모델이 사람들의 미래를 예측하는 데 사용될 수 있다는 것을 입증했습니다. 이러한 모델은 높은 정확도로 사망 시기까지 추정할 수 있다고 합니다.
Life2Vec 프로젝트: 인간의 삶을 예측하는 AI 모델
연구자들은 덴마크 통계국이 제공한 600만명의 덴마크인들의 건강 상태 및 노동 시장 참여 정보를 분석하기 위해 Life2Vec 모델을 사용했습니다. 이 모델을 훈련한 후, 그것은 데이터의 패턴을 학습하여 인격 특성 및 사망 시기와 같은 결과를 예측할 수 있습니다.
Nature Computational Science 저널에 발표된 전체 연구를 참고하세요.
👉 Using sequences of life-events to predict human lives
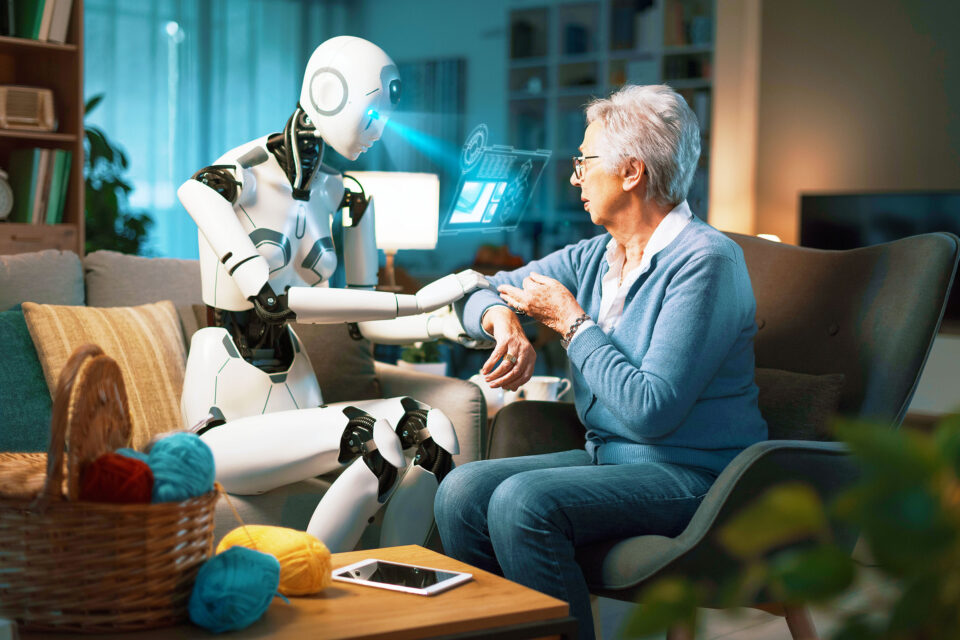
Transformer 모델: AI의 새로운 접근법
Life2Vec의 예측은 “4년 내에 사망”과 같은 질문을 다룹니다. 모델의 응답을 분석한 결과, 결과는 사회과학의 기존 연구 결과와 일치하는 것으로 나타났습니다. AI 모델은 출생 시간, 교육, 급여, 주택, 건강 등 다양한 요소에 대한 데이터를 대규모의 벡터 시스템에 배치합니다. 이러한 벡터 시스템은 데이터를 구성하는 수학적 구조입니다.
AI 예측의 함의
이러한 기술은 물론 인상적이지만, 모델의 데이터 보호, 개인 정보 보호 및 데이터 내 편견의 역할에 대한 윤리적 문제가 함께 제기됩니다. 질병 발생 위험이나 기타 예방 가능한 삶의 이벤트를 평가하기 위해 이러한 모델을 구현하기 위해서는 AI 예측결과에 대한 더 많은 정보와 통찰력이 필요합니다.
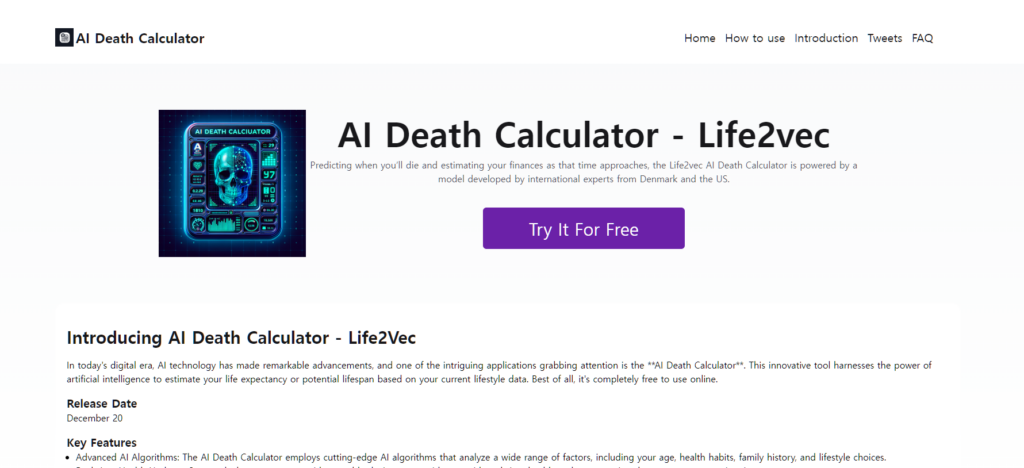
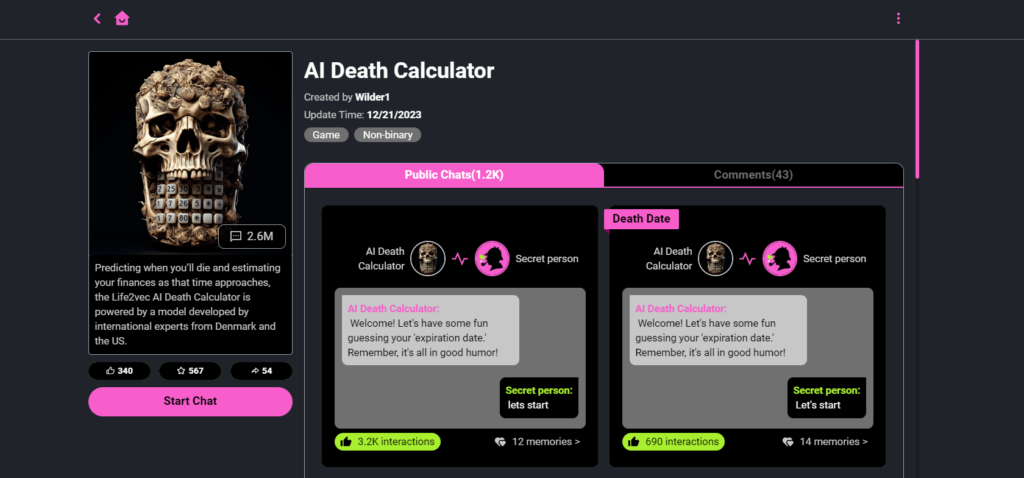
결론
Life2Vec 프로젝트는 인간 행동을 이해하고 예측하는 혁신적인 단계로, 의료부터 사회 정책에 이르는 다양한 분야에 대한 통찰력을 제공합니다. 인간의 삶의 복잡성을 더 깊게 탐구함에 따라, 가능성은 무한합니다. 앞으로는 이미지, 텍스트 또는 사회적 연결에 관한 정보와 같은 다양한 데이터 유형을 포함시키는 것이 다음 단계일 것입니다. 이러한 데이터 분석 및 인공 지능 예측의 발전은 미래 공중 보건 정책 및 개입을 설계하는 데 있어서 중요한 요소가 될 것입니다.
<영문버전>
주의 : 한국어 버전과 내용이 1:1로 상응하지 않으며, 독자의 이해를 돕기 위한 영문버전입니다. (번역본 아님)
Predicting Life Events with Machine Learning
Imagine if we could distill the essence of a person’s life into a series of numbers. Could we then accurately predict their future? This tantalizing prospect is no longer just a philosophical musing but a tangible reality, thanks to advancements in machine learning and data analysis.
In today’s digital age, our lives are increasingly documented and recorded. From online activities to financial transactions, a wealth of data is generated every day. Even in the public sector, institutions collect and store information on education, health, employment, and more. While much attention has been given to using health data for predictive modeling, there is a growing interest in leveraging broader life events to forecast future outcomes.
Traditional approaches to analyzing health records often rely on Natural Language Processing techniques, where words are converted into numerical vectors based on their context. Similarly, life events can be encoded into numerical representations, capturing key milestones such as education, taxes, and interactions with government services. These representations serve as valuable inputs for predictive models, enabling us to anticipate various life events such as retirement age, financial fraud, and even criminal behavior.
At the heart of this endeavor lies the Life2Vec project, utilizing data provided by Denmark Statistics containing sequences of events from millions of Danish residents. The goal is twofold: first, to develop models that accurately capture social behavior using a variety of machine learning techniques including recurrent neural networks, convolutional neural networks, and transformers. Second, it aims to assess the utility of these models in predicting life outcomes such as education levels, income, and employment history.
Given the sensitive nature of personal data involved, it says it prioritize the development of interpretable and secure models. Compliance with the latest legislative frameworks ensures data privacy and transparency, with models providing clear explanations for each prediction.
Life2Vec represents a groundbreaking step towards understanding and predicting human behavior, offering insights that could revolutionize fields ranging from healthcare to social policy. As it delves deeper into the intricacies of human life, the possibilities are limitless.
Pros and Cons Analysis:
Pros:
- Accurate Predictions: Machine learning models like Life2Vec can accurately predict various life outcomes, including education levels and income, which can be beneficial for individuals and policymakers.
- Potential for Social Impact: These predictive models have the potential to revolutionize fields such as healthcare and social policy by providing valuable insights into human behavior and societal trends.
- Interpretable Models: The emphasis on developing interpretable models ensures transparency and accountability, allowing stakeholders to understand the reasoning behind each prediction.
Cons:
- Privacy Concerns: The collection and analysis of personal data raise significant privacy concerns, especially regarding the use of sensitive information for predictive modeling without individuals’ consent.
- Risk of Bias: Machine learning models may inherit biases present in the data, leading to unfair or inaccurate predictions, particularly affecting marginalized communities.
- Ethical Implications: The use of predictive modeling in sensitive areas such as healthcare and criminal justice raises ethical questions about autonomy, fairness, and accountability, requiring careful consideration and regulation.
답글 남기기